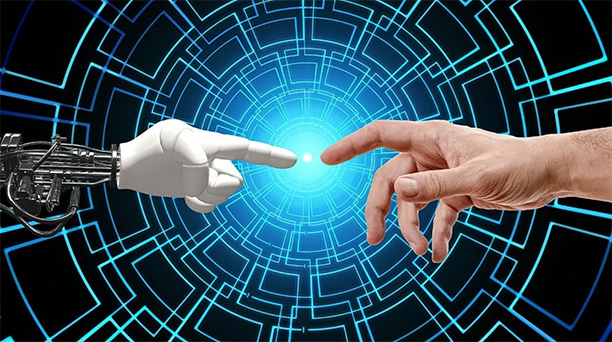
07 Mar Beyond the Hype: Evaluating AI Health Tech for Real Impact
Insight by: Debbie Lin
Introduction:
I’m a Venture Capitalist constantly evaluating exciting new AI health tech companies. I am also an Operator helping to build a precision medicine data company which had its beginnings as a pure diagnostics company. The field is booming, life sciences companies are morphing into data companies and tech companies are playing more in the space of healthcare and life sciences.
Recent announcements such as Isomorphic’s two major partnerships in January 2024 with pharmaceutical giants Eli Lilly and Novartis worth potentially $3 billion and more recently NVIDIA’s N Ventures.and Deerfield Management Company’s, 35 million dollar seed investment in Relation Therapeutics, a drug discovery company, headlined as the “ChatGPT for DNA” only add to the excitement and potential hype in AI based companies.
Asked how I separate true value from hype? Real impact comes from the foundation – the data and its clinical utility. Big funding announcements, don’t guarantee success.
The Challenge: Beyond the Latest LLM
As a VC, I face challenges in evaluating claims of having the “best” Large Language Model (LLM). LLMs are constantly evolving, making definitive superiority difficult. I also know how complex and challenging it is to create high quality data products that are clinically actionable.
Here’s my framework to assess any health tech company built on AI:
- Diving Deep into the Data Lake: Quality is King
Data is the lifeblood of AI health tech. My first step is examining the data lake, focusing on:
- Data Quality: The type (electronic health records, wearables, genomics) matters. It needs to be relevant, accurate, complete, and up-to-date.
- Focus on the Foundation: High-quality data is paramount for training the LLM. A weak data foundation can cripple even the most advanced LLM.
- Data Ingestion: How data enters the system is crucial, this signals how accurate the algorithms will be. I make mental note for frequency of data updates (real-time, daily, weekly) and robust security to protect sensitive patient information.
- Data Lineage: Understanding the data’s origin and journey is vital. I assess how the company tracks and documents data throughout its lifecycle. This transparency ensures responsible usage and helps identify potential biases.
- Building a Strong Infrastructure: From Chaos to Clarity
Raw data needs a strong infrastructure to become actionable insights. Here’s how I assess data management capabilities:
- Data Cleaning: Real-world data is messy. I evaluate methods for identifying and correcting errors, missing values, and inconsistencies.
- Data Collation and Structuring: Data from various sources needs integration and organization. I assess tools and processes for creating a unified and structured data lake, allowing the AI model to seamlessly analyze data from different formats.
- Data Organization and Accessibility: Even clean data is useless if inaccessible. I assess how data is organized and categorized for easy retrieval by different healthcare professionals (doctors, researchers). Intuitive organization enables efficient use of AI-generated insights.
- The Power of Usability: Turning Insights into Action
Ultimately, an AI health tech company’s value lies in its ability to drive positive change. Here’s how I assess application usability:
- Actionable Outputs: Does the AI application provide clear, actionable insights usable for clinical decisions or patient recommendations? In what time frame are the alerts or insights delivered? I look for concrete outputs that translate well into real-world healthcare settings.
- User-Centric Design: Is the application user-friendly for healthcare professionals with varying technical expertise? I prioritize applications with intuitive interfaces, clear visualizations, and seamless integration with existing healthcare workflows. User-friendly design encourages active use of the AI tool and its insights.
- The Data Moat: A Double-Edged Sword
Data exclusivity can be a competitive advantage. Ideally, the company should access exclusive data sources through partnerships with specific hospitals or research institutions. This proprietary data could be highly relevant and difficult for competitors to replicate, creating a “data moat.”
However, access to a specific data source doesn’t guarantee a moat. Regulations and privacy concerns often limit healthcare data sharing. Additionally, some data types, like electronic health records, might be readily available from various healthcare providers, reducing exclusivity.
Here’s what I also consider:
- Data Acquisition Methods: Exclusive partnerships or proprietary collection methods can strengthen the data moat.
- Data Accessibility for Others: Strict regulations and limited data sharing practices can make it challenging for others to replicate the data foundation.
- Transparency and Explainability: Understanding how the LLM arrives at its conclusions is essential. Companies that prioritize transparency in their LLM development inspire greater trust and confidence.
A Holistic Approach: Investing in the Future of Health
Successful AI health tech companies require more than cutting-edge technology. A high-quality data lake, robust infrastructure, and user-centric applications form the foundation for a company that can truly revolutionize healthcare. By carefully evaluating these components, I can invest in companies poised to make a lasting impact on patient outcomes and the future of healthcare.