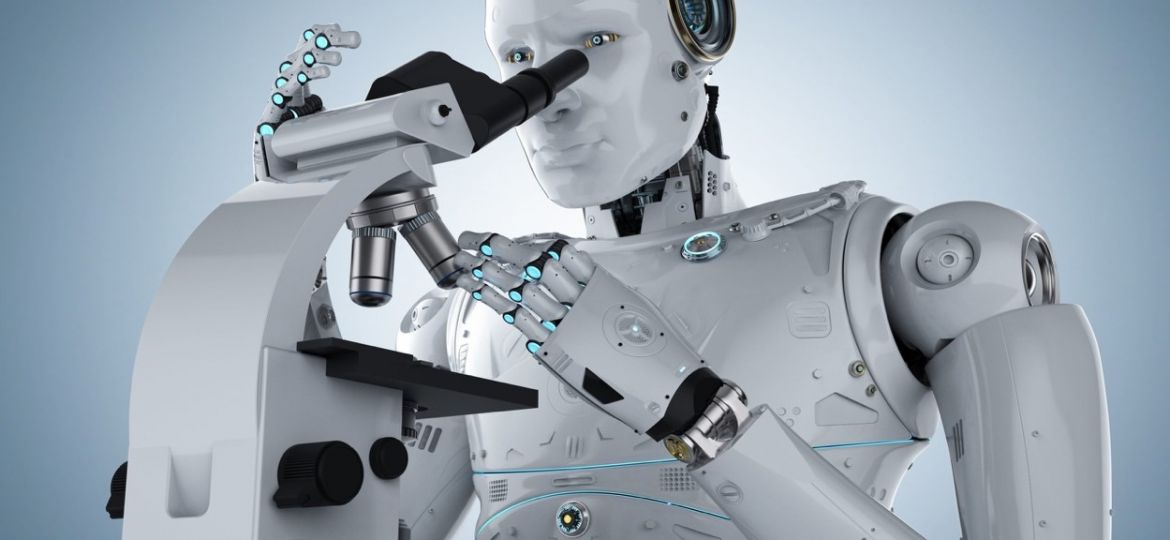
Insight by: Jon Warner
Life sciences companies have been increasingly investing in and exploring artificial intelligence (AI) technologies in recent years. In general terms, they see AI as having tremendous potential to help accelerate drug discovery, streamline clinical trials, improve patient outcomes, and reduce costs. But in what specific areas do Life Sciences companies see most applicability and usefulness? Here are 5 that are most often mentioned:
1. Drug Discovery
The drug discovery process is currently very long, costly, and inefficient. On average, it takes over 10 years and over $1Billion (some say the average is now nearer $3billion) to develop a new drug from initial research to final approval. AI shows promise in helping speed up and optimize various stages of drug discovery. For example, AI can analyze huge datasets to help identify new drug targets. Powerful machine learning algorithms can sift through molecular databases and scientific literature to pinpoint biological pathways and proteins most likely to be viable drug targets.
AI is also being used for virtual screening – analyzing vast libraries of chemical compounds to predict which ones are most likely to interact with and inhibit identified drug targets. This helps researchers focus their laboratory testing on the most promising candidates early on, avoiding wasted effort on compounds unlikely to become drugs. Some companies are developing “deep learning drug discovery platforms” that integrate target identification, virtual screening, and other early discovery tasks into end-to-end AI-powered workflows. This could significantly accelerate the timeline and lower the costs of drug discovery research.
2. Precision Medicine and Personalized Treatment
As genomics and other types of patient data become more widely available, life sciences companies see AI as key to realizing the promise of precision medicine. AI can uncover subtle patterns and correlations in huge and complex healthcare datasets that humans alone would miss. This helps identify biomarkers, develop diagnostic tools, and determine which patients are most likely to respond well or poorly to different treatments.
AI also enables more personalized treatment recommendations and monitoring. For example, AI models trained on a patient’s genetic profile and medical history can predict their risk for various diseases years in advance and recommend lifestyle changes or preventative measures. During treatment, AI combined with digital health tools can closely track patients, detect adverse events or worsening conditions early, and optimize dosing regimens in real-time based on individual responses. This level of personalized, data-driven care could transform patient outcomes.
3. Clinical Trial Optimization
Clinical trials are another area where life sciences companies see potential for AI to significantly improve efficiency and lower costs. AI can help with patient recruitment by better identifying eligible candidates from electronic health records. It can also assist with trial design by simulating different protocols and predicting enrollment rates, dropouts, and statistical power.
During trials, AI combined with IoT sensors and mobile or digital health apps enables remote and more frequent monitoring of patients. This improves safety, catches issues early, and reduces the need for in-person visits. AI can also automate tasks like adverse event detection from medical records and flag concerning safety signals for further review. Post-trial, AI expedites analysis of huge volumes of clinical trial data to accelerate regulatory review and approval. Overall, AI applications in clinical research aim to shave years and billions off development timelines and budgets.
4. Manufacturing and Supply Chain Optimization
Ensuring reliable, cost-effective production and distribution of drugs, medical devices, and other healthcare products is another area ripe for AI. Computer vision combined with machine learning can optimize manufacturing processes, catch defects early, and predict equipment maintenance needs to minimize downtime. AI also helps with demand forecasting, inventory management, and supply chain logistics to avoid stockouts or expiring products. This improves manufacturing efficiency, quality control, and customer satisfaction while reducing waste.
Some life sciences companies are implementing “digital twins” that model entire production facilities virtually. AI-powered simulations help optimize facility layouts, production workflows, and transportation routes in advance to maximize throughput. Real-time monitoring with AI then ensures physical operations match digital twins for continuous improvement. Overall, AI aims to transform life sciences manufacturing and supply chains into highly automated, data-driven processes.
5. Cost Reduction
Perhaps one of the biggest draws of AI for life sciences companies is its potential to help curb the skyrocketing costs of healthcare. By accelerating drug development, optimizing clinical trials, enabling precision medicine, and streamlining manufacturing/distribution, AI applications ultimately aim to bring treatments to market faster at lower prices. AI-powered tools also promise more efficient diagnostics, less invasive monitoring, and fewer unnecessary or duplicate tests/procedures.
Combined with electronic health records, AI enables proactive management of chronic conditions, early detection of health issues before they become severe/expensive to treat, and targeted interventions. If successful, these applications could help curb exorbitant healthcare spending on hospitalizations, procedures, and lost productivity due to illness. By improving outcomes and lowering costs across the entire drug development and delivery process, life sciences companies see AI as key to sustaining healthcare systems for the long term.
In summary, life sciences companies are actively exploring AI with the goal of revolutionizing drug discovery, clinical research, manufacturing, and healthcare delivery/costs. By leveraging vast amounts of data with advanced algorithms, senior executives broadly believe AI has potential to shave years and billions off development timelines, enable truly personalized medicine, optimize complex supply chains, and help curb unsustainable healthcare spending growth. While challenges remain, AI adoption in life sciences is accelerating with the promise of positively transforming patient outcomes and creating greater sustainability in the process.